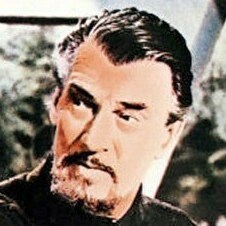
Perrow, Normal Accidents, and complex systems determinants
From comments to a post by @Joerg Fliege, preserved for easier retrieval.
Charles Perrow's model in Normal Accidents is Interactions vs. Coupling. This seems ... overly reductionist? Simple is good, too simple is not.
Breaking down Perrow's taxonomy, dimensions or factors I might apply. Ranges are generally from "easy" to "hard" in terms of successful control:
- Coupling flexibility: loose/tight
- Coupling count: low/high
- Internal complexity: low/high
- Threshold sensitivity: high/low
- Self-restabilisation tendency: high/low
- Constraints/tolerances (design, manufacture, operational, maintenance, training, financial): loose/tight
- Incident consequence: low/high
- Scale (components, mass, distance, time, energy (kinetic/potential), information, decision): low/high (absolute log)
- Decision or response cycle: long/short
- Environmental uniformity: high/low
- Environmental stability: high/low
- State determinability: high/low
- Risk determinability: high/low
- Controls coupling: tight/loose
- Controls response: high/low
- Controls limits: high/low
- Controls complexity: low/high
That's a bunch of factors, giving a complex model, but many of these are related. I see general parameters of complexity or arity, of change (itself complexity), of tolerances or constraints, of responses, of controls, of perception or sensing. These themselves are elements of a standard control or systems model.
update (learn)
^
|
state -> observe -> apply model -> decide -> act (via controls)
^ ^ ^ |
| / \ |
| system environment |
| |
+----------------------------------------------------+
Coupling is how the system relates to its environment and controls. Those couplings may also be sensors or controls.
Consequence refers to result of undesired or uncontrolled states. Relates strongly to resilience or fragility.
Internal complexity, threshold sensitivity, self-stabilisation, constraints, tolerances, and scale (a form or attribute of complexity) are all aspects of the system and its model. Consequence is a component of risk.
Decision cycle --- how rapidly responses must be made to ensure desired or controlled function --- is its own element.
Environmental uniformity and stability are exogenous factors.
State and risk determinability apply to observation and model, respectively. State is overt or manifest, risk is covert or latent. State is inherently more apparent than risk.
The controls aspects all all relate to how intuitive, responsive, limited, and complex control is. Controls mapping directly to desired outcome decrease complexity. Controls providing precise and immediate response likewise. High limits (large allowed inputs) increase control, low limits decrease it and require greater planning or more limited environments. Complexity ... my need some further refinement. Degrees of control mapping to freedoms of movement of the controlled system are useful, but complexity of interactions or in specifying inputs generally adds complexity.
On scale, I added the note "absolute log". That recognises that it's not simple large or small that is complex, but departure from familiar or equilibrium norms. A model isn't a smaller representation but a simplified one -- we model both galaxies and atoms. Starting with some familiar scale or equilibrium state, noting the orders of magnitude above or below that of a given system along various dimensions, and taking the absolute value of that, seems a reasonable first approximation of complexity of that system in that dimension.
Reducing my factors:
- System complexity: coupling, scale, internal complexity, stability, constraints, tolerances.
- Environmental complexity: uniformity, stability, observability, predictability.
- State determinability.
- Risk determinability.
- Model complexity, accuracy, and usefulness.
- Decision cycle: required speed, number of decisions & actions with time.
- Consequence: Risks. Result of undesired or uncontrolled state. These may be performance degredation, harm or damage to the system itself, loss of assets, reduced production or delivery, harm to operators, harm to third-party property, environmental degradation, epistemic harm, global sytemic risk.
- Controls: appropriateness, completeness, precision, responsiveness, limits, complexity.
That may still be too many moving parts, but I'm having trouble reducing them.
Perhaps:
- Complexity (state, system, environment, model, controls)
- Determinability (state, risk, consequence, decision)
- Risk (Or fragility, resilience, consequence?)
I'm not satisfied, but it's a start.
#complexity #CharlesPerrow #ComplexSystems #NormalAccidents #control #ControlTheory #SystemsTheory #Cybernetics #Risk #Manifestation #UnintendedConsequences #ManifestFunctions #LatentFunctions #RobertKMorton